SHARE
Digital Twin as a predictive tool for the industrial sector and product quality
Javier Flores, a Spanish engineer and technical director for R&D, participated in the Reusable mask challenge against COVID-19. This expert in manufacturing process automation shared with us his expertise on the Digital Twin in this article.
Digital Twin is one of the newly available technological trends in recent years for Industry 4.0. It can be defined as the virtual model of a process, product, or service through data obtained from sensors or automation.
This tool was born because of the market need of making industrial processes smarter and autonomous to adapt in real-time to changes in products, processes, and services and learn through experience. The Digital Twin covers the entire product lifecycle. It simulates, predicts, and optimizes the process, product, or service in industrial organizations.
Big companies opt for the inclusion of this powerful technological tool in their processes because they can obtain data from digital enablers to efficiently and accurately predict and manage any error or failure that may arise in their industrial processes. Thanks to these digital enablers, data can be obtained to facilitate the decision-making process for the optimization of processes, products or services. It is estimated that this tool helps increase efficiency by 10%.
For this reason, the Digital Twin must have a predictive focus and apply the hardware and software tools available in the market. Progress made in ‘Big Data’, ‘cloud computing’ or IoT simplify the introduction of Digital Twins in every kind of sector and production process.
The basic elements of the Digital Twin are the following:
The link between the Virtual Model and the Industrial Environment allows data control and analysis to predict inactivity periods through simulators. This creates new process optimization opportunities and, therefore, cost reduction.
The model of the 3D Digital Model must be a robust design, with the same parameters as the technical requirements we want to monitor. The correct execution of this electronic model will guarantee the reliability of the data captured in the simulations.
There are different programs to create this virtual model like SolidWorks, Catia V5, and AutoCAD. These powerful software programs allow the parameterization and analysis of the key control keys to make later changes in the entire value change without having to create new designs, which costs more money.
There are multiple sensors in the market for data gathering in a real environment. Flow control, vibrations, efforts, tensions, etc. are control parameters that will be used to predict the real environment and are valid for the simulation of potential errors that might occur over time.
A proper Data Management of the data captured during the manufacturing process must be treated with a predictive tool (Machine Learning) that boosts the necessary actions for the optimization of the Process, Product, or Service Lifecycle. Tools like BigML, Microsoft Azure, Tensor Flow, etc. add greater excellence to the treatment of data from the simulations of the Digital Twin, which allows for a thorough analysis of the information.
The ROI of the Digital Twin depends on the scope. The main benefits of this technology are the following:
In this case, the virtual model for the simulation has both the parts to be machined and the CNC programming, which estimates the times and the tool’s progress and revolutions for first data analysis.
Depending on the revolutions, progress and materials to be machined, the milling tools will be unequally worn out. The high cost of good milling cutters and the quality of the final product depend on this wear and the reached temperature. The thermal sensor will allow the heat transfer that occurs in the machined part during the grinding process. The cost of this sensor is very low and will serve as a learning parameter to avoid deformations during the process and optimize the machining process.
For this case study, the VERICUT software will act as a simulation tool to define the best parameters in the predictive process for the machining of parts that serve as examples for the simulation. Once this data has been simulated, the sensors will verify them on-site. These are the sensors that will be used for the verification:
This analysis enables the prediction, according to the data from the simulator, the progress, and revolutions needed per material that better adapts to the described requirements for the optimization of the tool wear.
Once the parameters have been defined, will go on to verify them on-site, getting real data that, through learning, will further optimize this process. Maintenance and halts will be reduced thanks to a better performance of the tool and the process and, therefore, there will be a cost reduction.
The estimated costs of this example will vary depending on the resources and the expected tolerances for the final product. For this example, the investment costs are estimated at 24,000 € - 30,000 €, given the high cost of the high-accuracy verification sensors (software license not included).
If you want to read more about automation and artificial intelligence, check out ennomotive’s blog and discover what ennomotive can do for you.
Join the engineering community
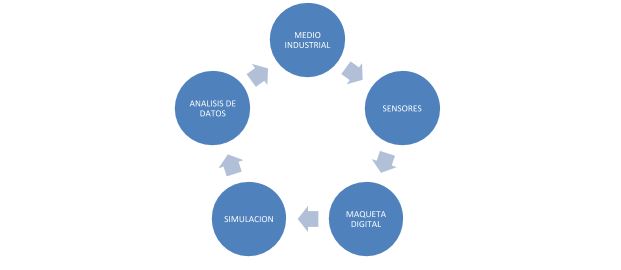
- Predictive and Corrective Maintenance
- Reduction in production halts
- Optimization of the line costs
- Energy efficiency
- Product quality
- Engineers hours for the design of the parameterized 3D model
- Simulation software adapted to the technical requirements
- Data gathering sensors
- Data engineering for analysis (Machine Learning)
- Communication and Monitoring platform
CASE STUDY: Optimization of the Machining process in a Machining Center
This simple example aims to optimize the milling process in a machining center. The goal is to reduce the number of tool changes due to the wear of the milling cutter, optimize the energy consumption to become more energy-efficient, and achieve that the machining center has the best performance for greater durability. The control parameters to achieve these goals are the following:- Progress of the tool
- Revolutions of the tool
- Milling cutter
- Material to be machined
- Electricity consumption
- Vibrations
- Dimensional verification
- The temperature of the machined material
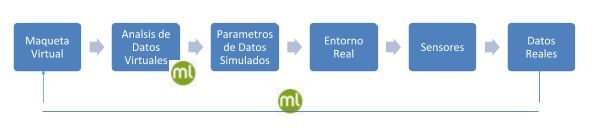
- PC Industrial i7 Schneider ( 700 €)
- WINDOWS 10 PRO 64 BITS OEM ( 150 € )
- Software and Hardware for the verification of the TCP tool wear.
- Temperature sensor (120/200 €)
- Power control POWERMETER PM5320 Schneider (700€)
- 3D verification per part scanning. 3D laser profile meter (6,000€). Other technologies are also available in the market.
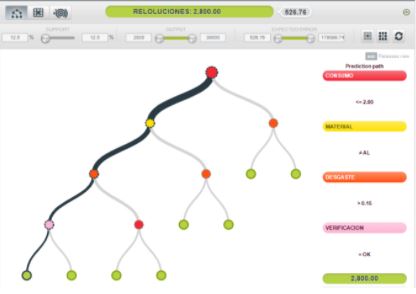